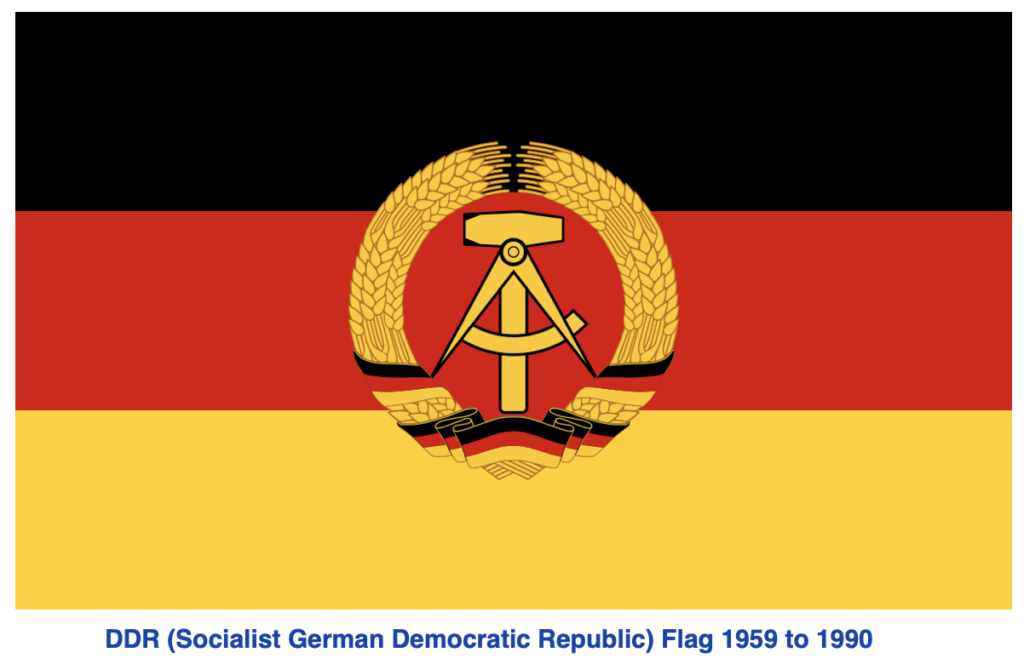
By Alex Dortzbach –
Media lies very near the heart of a culture in recording values. It can help create a cultural lexicon, build or reinforce a set of common ideals, and quicken the spread of new ideas. A hugely-insightful book, The Race Against the Stasi (2014) by journalist Herbie Sykes tells the story of a cyclist in the East German DDR / GDR who defected to West Germany. Reviews describe the book as “gripping”, “an incredible story”, “like the best espionage novels…cannot be put down” and it won much added acclaim besides being Cycling Book of the Year. Sykes chronologically documents the story of Dieter Wiedermann and those close to him through interviews, now-declassified East German STASI (Ministry for State Security) files, photographs, and East German newspaper articles. I would like to thank Herbie Sykes for answering all of my questions about his book and for his astute clues about the East German DDR / GDR government’s published Neues Deutschland archived articles.
Neues Deutschland, the official party newspaper of the Socialist Unity Party (SUP) in the German Democratic Republic (GDR/DDR), is really no different than other government-controlled media. From 1946-1990, it was a vitally important organ of the SUP, and had a circulation of 1.1 million in 1989. This East German newspaper was not only a way to spread (as well as influence and control) information; reading it can also provide critical insight into the state and values of a culture. Can these values be analyzed and quantified, possibly using emotive language?
In this article I use machine learning to analyze the emotion and tone in articles from Neues Deutschland. This will demonstrate how the tone of articles has evolved over time and highlight the impact that different emotions can have on the text. Both emotion and tone are key factors in shaping disinformation, as I’ll illustrate in the context of the Berlin Wall.
Method
Sentiment analysis is a way of using machine learning techniques to analyze the tone or emotion of a work of text. For example, if the text contains the sentence “I love cats”, the text will be evaluated to have a more positive tone. This same technique can be used to identify different emotions being expressed in a text. In my evaluation of the Neues Deutschland Articles, I used machine learning to perform this sentiment analysis.
I selected articles from Neues Deutschland (ND) using a random date generator. My representative sampling size dataset contains 87 front-page articles–all from different issues of the newspaper–written between 1946 and 1990. For statistical parameters, the variables I employed are x = number of ND articles), y = range of emotions analyzed. If I had a z variable, it has to be an as yet unknown factor regarding all the possible emotive occurrences in the ND texts.
Some other statistical parameters include (line of best fit): average sentiment value = 0.498; coefficient = 0.00797; intercept = -15.207; standard deviation = 0.301; r squared = 0.109. The five emotions analyzed (x) are joy, anger, fear, surprise and love. As mentioned above, my (y) number of ND articles is 87. But total possible emotive occurrences in the ND texts used (a hypothetical z variable) are impossible to know because the pre-built machine learning model I used only detected the above 5 emotions. The machine learning model I used also quantifies the emotive strength but does not point to individual instances which are emotive.
I used Google Translate to translate the text to English. This allowed me to use specific models to analyze emotion which did not exist for the German language. There is precedent for using machine-translated text effectively for linguistic models (de Vries et al., 1), although they are performing a different technique of sentiment analysis that does not rely on machine learning. A limitation of machine translation such as Google Translate is how it handles idiomatic language. With limited context, Google Translate will sometimes translate idioms literally, which affects the tone of the text. This is a raw factoring variable that could skew a sentiment analysis model. However, the dataset of articles I am using has limited use of idiomatic language. This is representative of the larger corpus of Neues Deutschland articles. To mitigate other effects that translating the data may have, I will use comparative sentiment scores rather than the raw sentiment scores. This provides that if the machine translation shifts the sentiment, that shift will be consistent across the dataset. Additionally, because I am calculating sentiment scores for large pieces of text, an instance of mistranslation will have a small if not negligible effect on the final sentiment score.
I used two different models to run my sentiment analysis. They are both related to the DistilBERT model, which is a smaller version of Google’s BERT model for sentiment analysis. The model I used to detect a positive or negative tone is a version of DistilBERT which was fine-tuned on the Stanford Sentiment Treebank 2 dataset (SST-2). The SST-2 dataset consists of 11,855 single sentences extracted from movie reviews (Socher). The model I used to evaluate emotions in the text was a version of DistilBERT trained on a Twitter sentiment analysis dataset to identify five emotions (Savani).
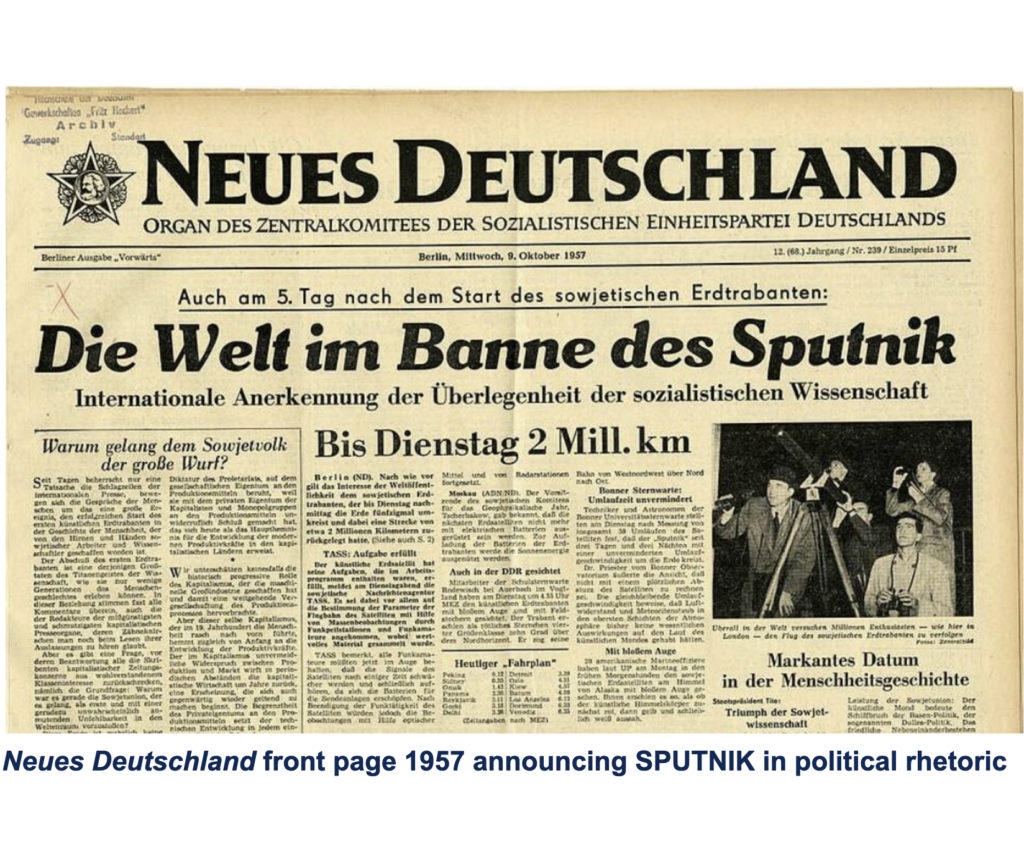
Sentiment Polarity in Neues Deutschland
The tone of newspaper articles has been shown to significantly influence readers’ opinions. A study of the 2005 UK General Election Campaign shows that the impact of tone in a newspaper is significant and polarizing, even towards entities not mentioned. A positive tone in an article towards the Labour party resulted in a positive sentiment score towards Labour in readers, but also a negative effect on the scores for the Tory party (Brandenburg 454). The inverse is true as well; a negative tone towards one entity can have a positive effect on its opponent, even if it is not explicitly named. While the Neues Deutschland articles do not focus on political parties, West Germany is constantly set up as an opponent to the DDR / GDR. The tone of the articles about both West Germany and the DDR / GDR would have a significant impact on how both nations are viewed by its readers.
To determine the valence, or how positive or negative the tone is, I ran each sentence of the article through the DistilBERT model. Each sentence was assigned a score between -1 and 1 corresponding to how negative or positive the tone of the text is. Calculating the mean sentiment of each article resulted in the following:
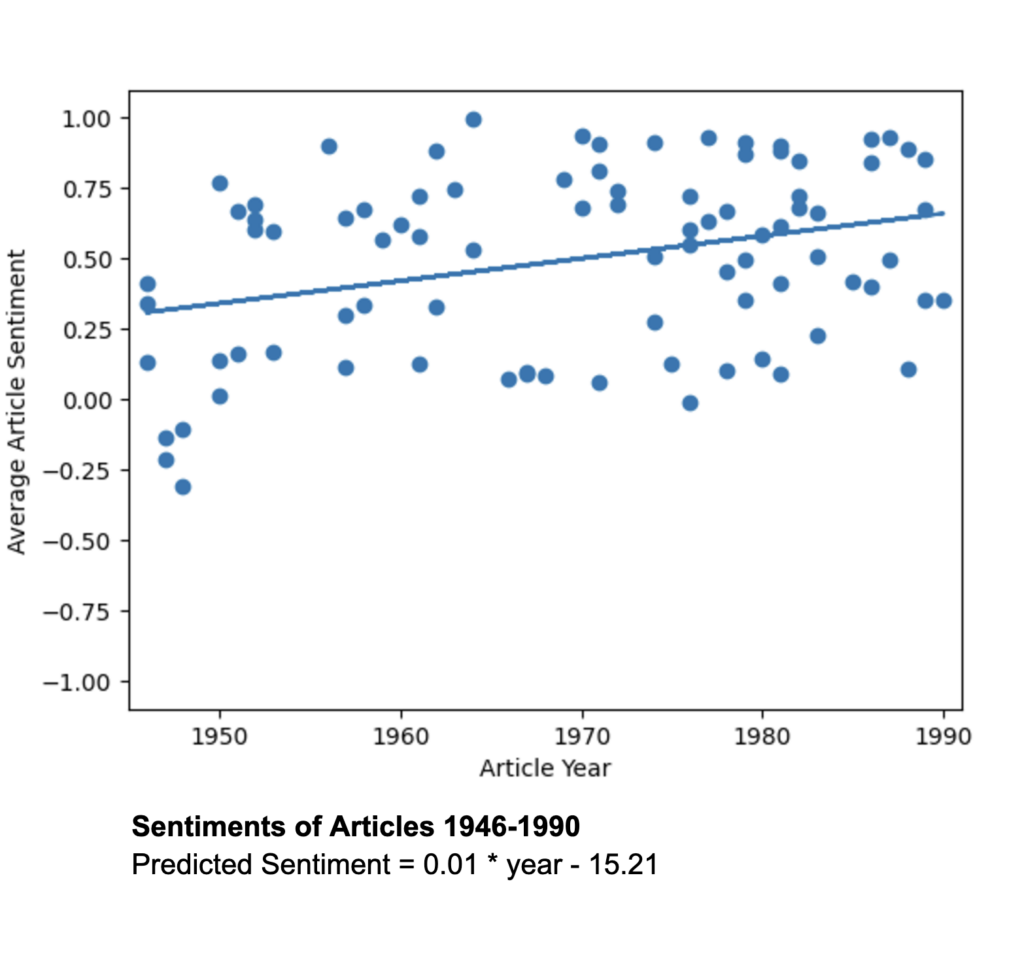
There is a slight positive trend in the sentiment of the articles between 1946 and 1990. However, the coefficient of determination of 0.11 is quite low, indicating that while the line of best fit does indicate an upward trend in sentiment, it is not an effective predictor of article sentiment.
One element which could be creating extra variance in this dataset is the frequent use of country names in Neues Deutschland. The DistilBERT model is heavily biased by country names, and as can be seen in the figure below, the word “Germany” heavily skews the data towards a negative sentiment (called “HuggingFace” in the model).
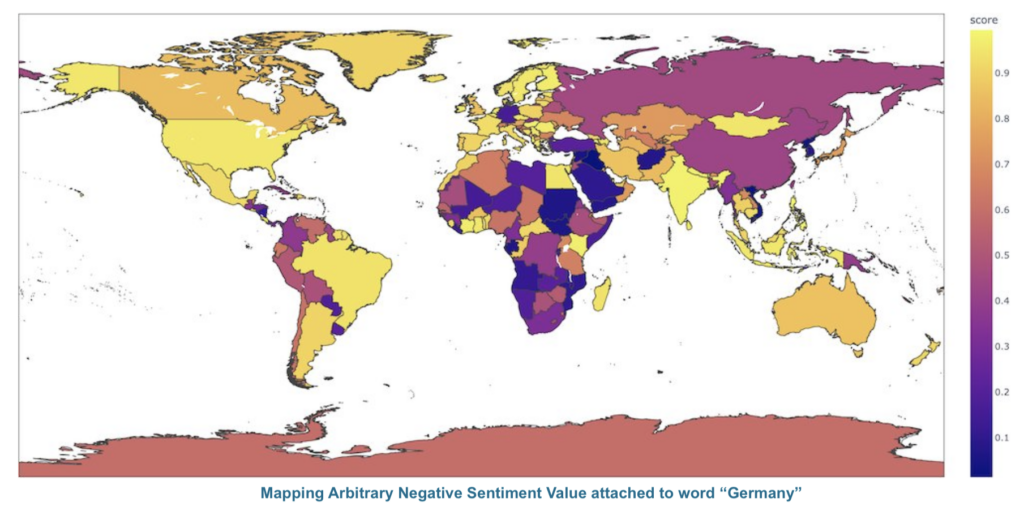
The solution to this bias would be to mask all country names in the articles before running sentiment analysis, so that all country names are processed as the equivalent neutral words in the model.
Emotion in Neues Deutschland
Describing a tone as positive or negative is a useful tool, but can only go so far in analyzing the content of a piece of text. “Negativity” is a nonspecific descriptor when it comes to a newspaper article; it could mean anything from a reporter writing about a negative current event to expressing disapproval of a policy or politician. Elation and contentment are both “positive” emotions, but describe very different states.
This is especially true for fear and anger; both can describe a negative tone but have very different effects on a reader. Anger tends to decrease a person’s perception of risk, and is politically motivating. Fear has the opposite effect, increasing a person’s risk perception and immobilizing them. An example of this can be seen after 9/11; Huddy, Feldman, and Cassese (2007) found that anxious people were less likely to support the Iraq war, while angry respondents were more likely to support the war (Soroka et al. 111). Understanding the emotions used in Neues Deutschland can provide insight into the mindset and intentions of people of the GDR.
To determine the emotional sentiment scores, I ran each sentence of the article through a DistilBERT model which was pre-trained to classify joy, love, surprise, sadness, fear, and anger in text. Each sentence was assigned a score between 0 and 1 representing the proportion of that emotion in the text, and all of the emotion scores sum to 1. Calculating the mean sentiment across all articles for the each of the emotions gives the following:
Joy: 0.440
Anger: 0.3664
Fear: 0.108
Sadness: 0.0630
Love: 0.0149
Surprise: 0.00759
My initial hypothesis was that fear and anger would outweigh the other emotions. Yet, most of the articles I sampled have either a joyful or angry tone. It is notable how low the sentiment value for fear is across the dataset–its average score was only marginally higher than that of sadness. The sentiment values for sadness, love, and surprise are all quite low.
The strong presence of anger and joy in the Neues Deutschland (ND) articles suggests a few things. Both are powerful, persuasive emotions. Joy, the dominant positive emotion, is tied to optimism, while anger, the main negative emotion, creates agitation rather than pessimism. Fear, another negative emotion, appears rarely in the dataset. This emotion-driven rhetoric, consistent from 1946 to 1990, shows that Neues Deutschland sought to rally readers against state enemies and replace any anxiety or dissatisfaction with optimism about the DDR / GDR.
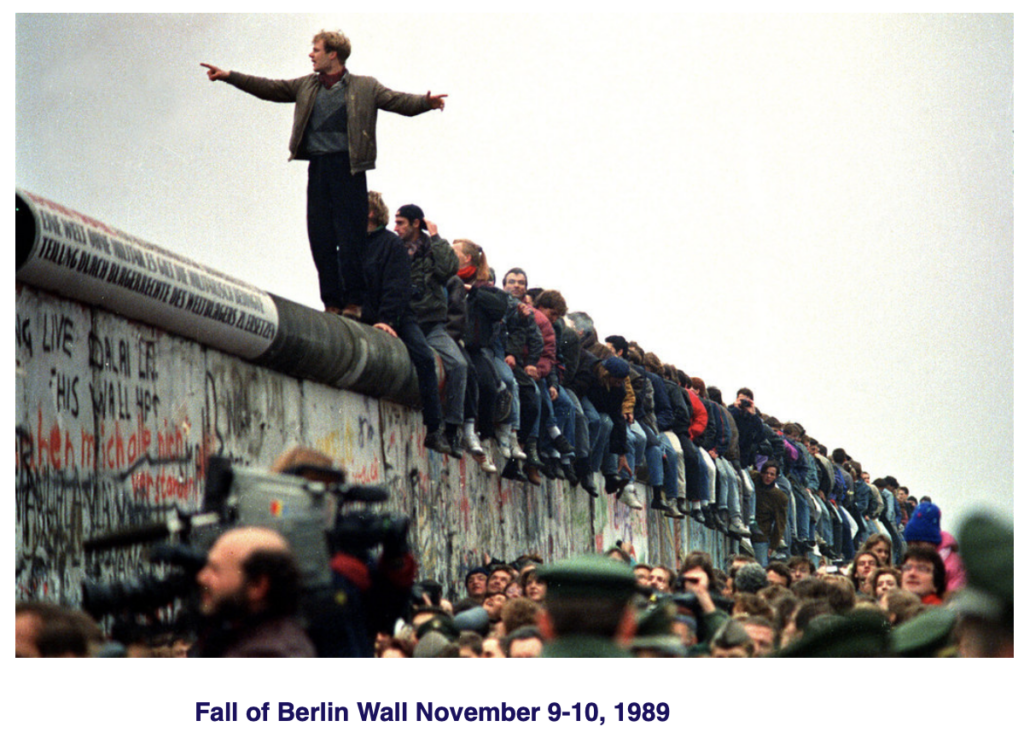
Fall of the Berlin Wall
The Berlin Wall fell the night of November 9, 1989. The front page of Neues Deutschland on November 10th was consumed with the recent Communist Party conference. Future intentions of the party, lists of event speakers, and internal structure of the party fill the next many pages of Neues Deutschland.
The article on the 11th would be the first which could have been written after the Berlin Wall fell. The front page article on November 11 seems to describe the Peaceful Revolution in Berlin and the future of the GDR:
“The German Democratic Republic is on the move. A revolutionary popular movement has set in motion a process of serious upheaval… It took peaceful mass protests by the population, the expression of will by many political organizations, the constructive work of church circles and the growing pressure from the base of our own party, as well as a learning process in the party leadership, to break up the rigid political structures and initiate the first steps towards a change.”
Though the ND articles cover the same event — the Communist Party conference — the tone of the November 11 article is notably more positive and joyful compared to the previous day’s. Both articles are about the same topic, but the ND article on the 11th has a more positive sentiment and exhibits a more joyful tone than the article on the 10th.
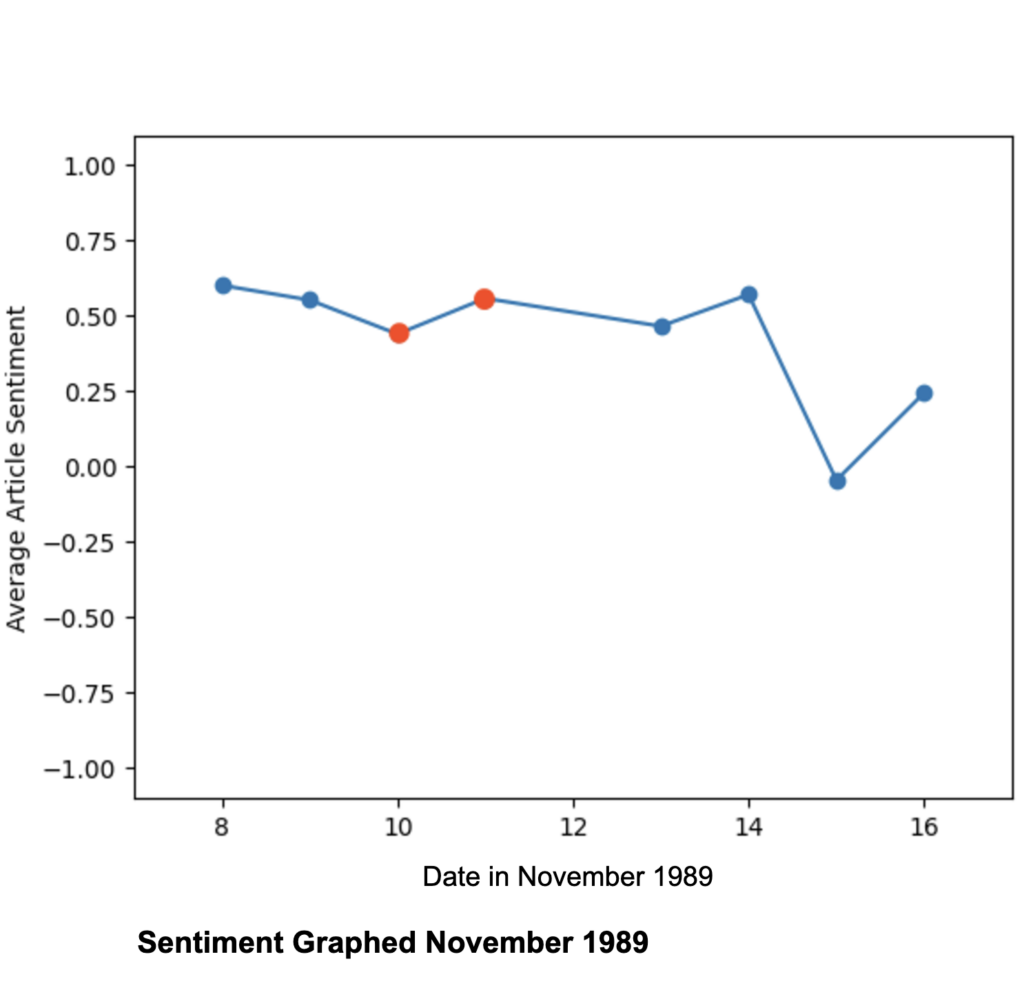
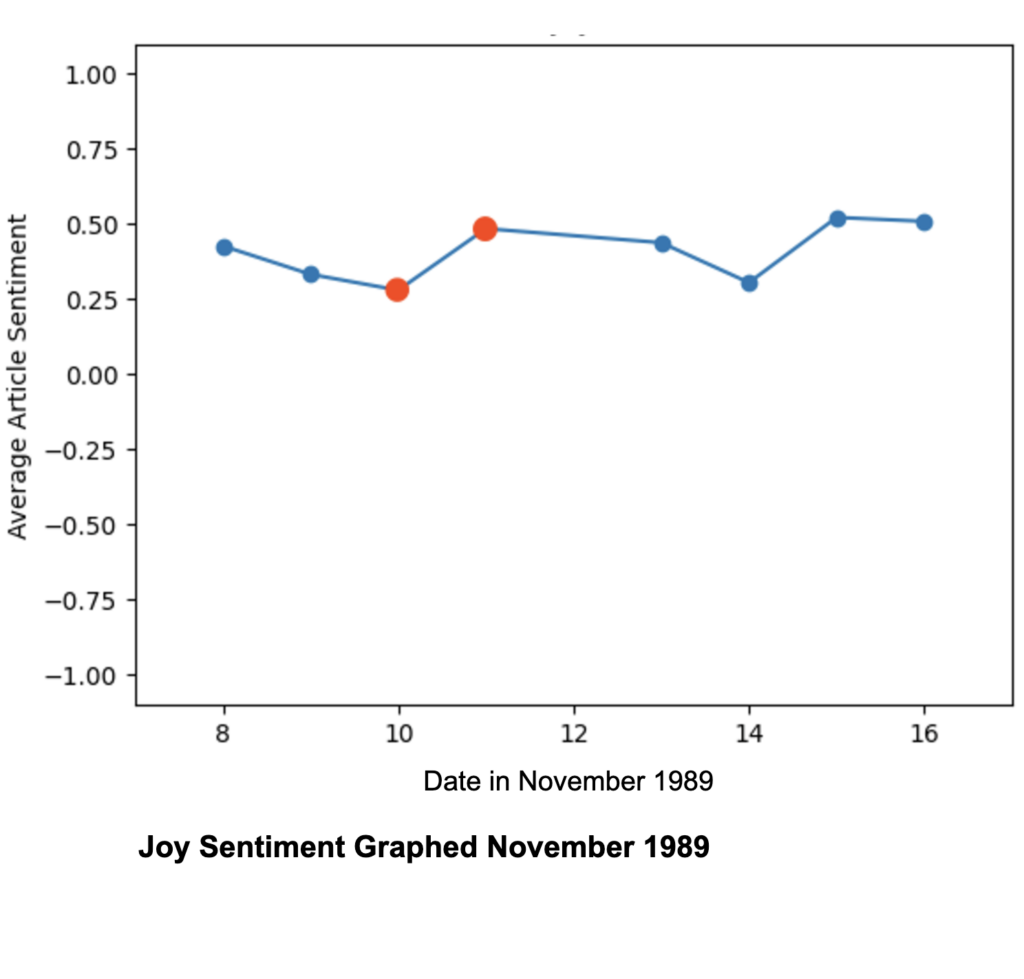
Despite its outward focus, the ND rhetorical language hints at the Wall’s fall, suggesting an effort to shift attention away from it. Both of these things point towards the use of rhetorical manipulation to divert attention from the fall of the Berlin Wall. This manipulation of tone and sentiment demonstrates how emotion plays a key role in disinformation. Sentiment analysis can therefore be a valuable tool for detecting bias in media and propaganda.
Conclusion
The analysis of Neues Deutschland articles using sentiment analysis has revealed how emotion and tone were strategically employed to influence public perception in the German Democratic Republic. Joy and anger were frequently used–emotions are both motivating; one in the positive sense and the other in the negative. The example of the Berlin Wall’s fall in November 1989 highlights how Neues Deutschland manipulated emotional tone in the wake of such a monumental event. Despite the dramatic political upheaval signaled by the Wall’s fall, the newspaper’s coverage quickly pivoted to focus on party affairs and future plans, using positive language to maintain control over the narrative and direct attention away from the political shift that was already underway. This strategic manipulation of tone underscores the importance of the newspaper in shaping public sentiment during critical moments of change. There is an extensive archive of Neues Deutschland – even after the GDR fell. Interestingly the newspaper is still in print today under the same name, though its circulation has dwindled dramatically to 16,028 readers – 1% of its previous readership – as of 2022. By studying the rhetoric of Neues Deutschland, we gain valuable insights into the circumstances, aims, and eventual dissolution of the German Democratic Republic, providing a unique perspective on how media was rhetorically used to shape public sentiment and maintain political power, no doubt profoundly relevant to the very similar ongoing role of politicized media in 2024-25.
Works Cited
Brandenburg, Heinz, and Marcel Van Egmond. “Pressed into Party Support? Media Influence on Partisan Attitudes during the 2005 UK General Election Campaign.” British Journal of Political Science, vol. 42, no. 2, 2012, pp. 441–63. JSTOR, http://www.jstor.org/stable/41485691. Accessed 31 Dec. 2024.
Devlin, Jacob, and Ming-Wei Chang. “Bert: Pre-Training of Deep Bidirectional Transformers for Language Understanding.” Google Research, research.google/pubs/bert-pre-training-of-deep-bidirectional-transformers-for-language-understanding/. Accessed 31 Dec. 2024.
de Vries, Erik, et al. “No Longer Lost in Translation: Evidence That Google Translate Works for Comparative Bag-of-Words Text Applications.” Political Analysis, vol. 26, no. 4, 2018, pp. 417–30. JSTOR, https://www.jstor.org/stable/26563863. Accessed 31 Dec. 2024.
Huddy, Leonie, et al. “The Political Consequences of Perceived Threat and Felt Insecurity.” The Annals of the American Academy of Political and Social Science, vol. 614, 2007, pp. 131–53. JSTOR, http://www.jstor.org/stable/25097965. Accessed 31 Dec. 2024.
HuggingFace Canonical Model Maintainers. “Distilbert/Distilbert-Base-Uncased-Finetuned-SST-2-English · Hugging Face.” Distilbert/Distilbert-Base-Uncased-Finetuned-Sst-2-English · Hugging Face, huggingface.co/distilbert/distilbert-base-uncased-finetuned-sst-2-english. Accessed 31 Dec. 2024.
Kleppen, Eric. “What Is Sentiment Analysis? What Are the Different Types?” Built In, 3 Mar. 2023, builtin.com/machine-learning/sentiment-analysis.
Sanh, Victor, et al. “Distilbert, a Distilled Version of Bert: Smaller, Faster, Cheaper and Lighter.” arXiv.Org, 1 Mar. 2020, arxiv.org/abs/1910.01108.
Sarotte, Mary Elise. “It Wasn’t Reagan Who Made the Wall Come Down.” Wall Street Journal, 31 Oct. 2014, www.wsj.com/articles/book-review-the-collapse-by-mary-elise-sarotte-1414788690.
Savani, Bhadresh. “Bhadresh-Savani/Distilbert-Base-Uncased-Emotion · Hugging Face.” Bhadresh-Savani/Distilbert-Base-Uncased-Emotion · Hugging Face, huggingface.co/bhadresh-savani/distilbert-base-uncased-emotion. Accessed 31 Dec. 2024.
Sykes, Herbie. The Race against the Stasi: The Incredible Story of Dieter Wiedemann, the Iron Curtain and the Greatest Cycling Race on Earth. Aurum Press, 2014.
Wilder, Charly. “East German Papers Offer Glimpse of History.” SPIEGEL International, https://www.spiegel.de/international/germany/east-german-newspapers-digitized-and-posted-online-a-908216.html.
Wolf, Thomas, et al. “Huggingface’s Transformers: State-of-the-Art Natural Language Processing.” arXiv.Org, 14 July 2020, arxiv.org/abs/1910.03771.
Würz, Markus. Sputnik-Schock. Living Museum Online, Foundation House of History of the Federal Republic of Germany, www.hdg.de/lemo/kapitel/geteiltes-deutschland-gruenderjahre/kalter-krieg/sputnik-schock.html. Accessed 25 Jan. 2025.
Zefys Zeitungsinformationssystem, Staatsbibliothek zu Berlin, zefys.staatsbibliothek-berlin.de/list/title/zdb/2532889X/. Accessed 31 Dec. 2024.